In‐hospital clinical artificial intelligence (AI) encompasses learning algorithms that use real‐time electronic medical record (EMR) data to support clinicians in making treatment, prognostic or diagnostic decisions. In the United States, the implementation of hospital‐based clinical AI, such as sepsis or deterioration prediction, has accelerated over the past five years,1 while in Australia, outside of digital imaging‐based AI products, nearly all hospitals remain clinical AI‐free zones. Some would argue this is a good thing, both prudent and sensibly cautious given the wide ranging ethical, privacy and safety concerns;2,3 others contend our consumers are missing out on important interventions that save lives and improve care.4,5 In this perspective article, we argue that in‐hospital clinical AI excluding imaging‐based products (herein referred to as “clinical AI”) can improve care and we examine what is preventing clinical AI uptake in Australia and how to start to remedy it.
Most clinicians know about the failures of the Epic Sepsis Model in the US.6,7 The AI model missed 67% of septic patients (prevalence 7%) and generated alerts for 18% of all hospitalised patients and, when compared across 15 hospitals (six without the prediction tool), there were no improvements in antibiotic treatment rates or patient outcomes. Other similar high profile failures litter both journals and general news outlets, for example:
- Google's Verily Health Science product to detect diagnostic retinopathy in Thailand, where 21% of images were rejected by the system because of different lighting conditions on site and different patient preparation procedures;8
- Stanford's evaluation of skin cancer detection products revealing significant effectiveness drops between light and dark skin patients;9 and
- IBM's abject failure to get Dr Watson off the ground after spending US$5 billion, with clinicians “wrestling with the technology rather than caring for patients”.10
Bad news makes for memorable news, but what is missed in the burgeoning number of journal articles on clinical AI11 is the myriad success stories. In a recent systematic review of implemented sepsis prediction models worldwide, eight systems were installed across more than 40 hospitals; five systems reported mortality, of which all reported reductions and two significantly reduced.5 We did a similar investigation of implemented clinical deterioration prediction systems which revealed similar results.12 The implications for the application of AI to care is wide ranging. A study published in 2021 reported over 20 different implemented applications of AI in clinical settings including, among others, prediction models for stroke, hypertension, venous thromboembolism and appendicitis.1 Of these, 82% were implemented in the US and none in Australia.
Australian health care seems impervious to the alure of AI. We searched each Australian state and territory's public health care websites and found just two clinical AI stories. Across a network of clinicians in a national AI working group, only one hospital was known to have an AI trial underway. As far as we are aware, there is no clinical AI implemented across Queensland Health despite having Australia's largest centralised EMR system, which could make large‐scale AI feasible. In stark contrast to the number of implemented AI systems, AI research abounds, with nearly 10 000 journal articles published each year across the world.11 Why is clinical AI not translating to clinical practice in Australia?
There are many common reasons cited for a lack of AI uptake within health care, including lack of clinician trust in unexplainable models,2 data privacy concerns from consumers,13 health inequity concerns due to underlying data biases,2,13 and underdeveloped or absent government regulation.14 But these reasons are similarly applicable worldwide and yet do not prevent other countries from translating AI from the laboratory to their clinical practice. Perhaps the World Health Organization's recent caution can provide a clue, “Precipitous adoption of untested systems could lead to errors by health‐care workers, cause harm to patients, erode trust in AI”.15
What does an “untested system” mean, or, more importantly, what constitutes a tested AI system, that is, one that health authorities would be willing to implement within their hospitals? As far as we are aware, no such framework for the safe introduction of AI into clinical practice exists in Australia. However, it does overseas. To explore this question further, we conducted a scoping review of clinical AI implementation guidelines, standards and frameworks and identified 20 published articles since 2019 from seven countries.16 We found there were common stages to AI implementation to ensure the safe, effective and equitable introduction of AI into clinical practice. Although these stages vary, they generally always include a stage for problem definition to check that AI is needed and possible (stage I); retrospective (in silico or laboratory) evaluation to ensure that AI meets minimum performance requirements (stage II); prospective evaluation, often called a silent trial using real‐time EMR data, to evaluate in an environment with zero patient risk the clinical utility, live AI performance, alert functionality, user interface design and the data quality and latency impact (stage III); a pilot trial to assess patient safety and clinical workflow integration (stage IV); and a larger clinical trial or rollout (stage V).
Knowing these stages provides a helpful framework for thinking about a multistaged approach to AI testing but does not provide sufficient detail for practical use. However, for evaluation stages II–V, there exist international reporting standards (TRIPOD,17 DECIDE‐AI,18 CONSORT‐AI19) that prescribe detailed elements to promote rigorous and transparent evaluation of AI interventions, and these standards are widely known and used by the international clinical research community. We integrated these standards into each stage of implementation and, together with broader findings from 20 other international frameworks, derived an end‐to‐end clinical AI implementation framework called SALIENT (Box 1).16 SALIENT is the only framework with full coverage of all reporting guidelines so that it may provide a starting place for establishing that AI is tested and suitable for implementing into Australian health care.
Although we contend that adopting a framework for testing and implementing AI is going to be necessary if Australia is to introduce AI safely into clinical practice, we do not suggest this is the major reason for low AI uptake. What it has highlighted though, is a gap in Australia's health care infrastructure that is preventing the translation of AI into clinical practice. There is a reason that almost all AI research is conducted at stage II (retrospective evaluation of AI): it is relatively easy to conduct on any ethics‐approved historical dataset at low cost and journals have been keen to publish the findings of such studies. A counterpoint to this is the stage III prospective or silent trial (Box 1, red dashed box). Prospective trials require live or near‐live access to EMR data, including all the legislated and necessary data governance hurdles to access these identifiable data, and, most importantly, an information technology (IT) infrastructure that supports live trials, which typically take from three to six months.5
Suitable prospective trial infrastructure is probably the most important requirement for the safe introduction of AI in Australian hospitals. Our public health care organisations have been equipped with EMRs relatively recently — over the past decade, however, none are funded or resourced to create such an infrastructure. Conducting a silent trial for just a single AI intervention requires a technology solution (Box 2) and expertise to:
- stream or batch potentially millions or billions of patient transactions each second that can be used by the AI model;
- develop and run a data pipeline that can transform the live data for each variable for each patient, including data cleaning, imputation, aggregation and normalisation, so that it can be fed into the AI model;
- develop and integrate an AI model to perform inference and a place to report results;
- label patient outcomes (eg, whether or not the patient has sepsis);
- evaluate algorithm performance results; and
- orchestrate trials with auditable results over weeks and months.
Such infrastructure exists in the US.20 However, as far as we are aware, it does not exist in Australia, except where constructed for bespoke AI trials, such as the predictive sepsis trial in New South Wales Health (unpublished data). The costs to develop and run such infrastructure are prohibitive for individual publicly funded health care organisations and, further, such organisations struggle to find the experts necessary to build it.
Without the capacity to conduct prospective trials, health care organisations are missing the most important patient risk‐free stage in the implementation process that assures them that AI interventions can be safely run on their IT systems, within their hospitals and with their patients. AI algorithms are typically developed and evaluated on different datasets to those of the target hospital sites. This means that changes to clinical workflows, presenting patient conditions, data quality levels and patient demographic distributions can significantly affect algorithm performance. For example, AI algorithms developed on large city populations may perform poorly for hospitals in rural and remote areas, further perpetuating poor health outcomes for underserved and marginalised patient cohorts. Without this evaluation checkpoint, the AI remains untested. We argue this is one of the major reasons for the slow or absent uptake of AI within Australian hospitals today.
To resolve this impasse, public funding outside of health care organisations’ budgets is required to develop this infrastructure. A standardised prospective evaluation infrastructure should plug into a range of EMRs (eg, Cerner, Epic), support most hospital‐based AI interventions, and be able to be deployed within each health care organisation's firewalls. Such an infrastructure, coupled with a standardised AI implementation framework, could provide health care organisations with the tools they need to comprehensively evaluate the AI and with the confidence they need to move beyond retrospective studies and implement well tested AI into clinical practice.
Box 1 – Abridged version of the SALIENT end‐to‐end clinical artificial intelligence (AI) implementation framework*
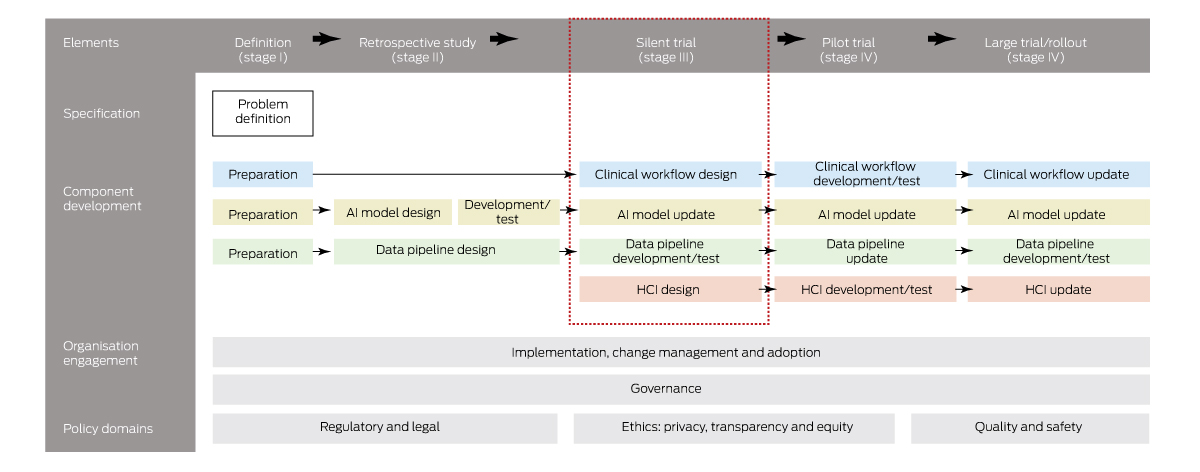
HCI = human–computer interface. * The coloured boxes refer to AI solution components (see Component development section): blue = clinical workflow; yellow = AI model; green = data pipeline; red = HCI. Source: Figure adapted from van der Vegt et al.16
Provenance: Not commissioned; externally peer reviewed.
Open access:
Open access publishing facilitated by The University of Queensland, as part of the Wiley ‐ The University of Queensland agreement via the Council of Australian University Librarians.
Anton van der Vegt is supported by the Advance Queensland Industry Research Fellowship granted by the Queensland Government. The funder played no role in the writing or publication of this study.
No relevant disclosures.