The known: More than 80% of strokes could be prevented by effective management of cardiometabolic risk factors. However, little is known about their management in Australian general practices for the secondary prevention of stroke.
The new: About three in four people who survived stroke or transient ischaemic attack and were managed in 383 Victorian general practices attained guideline‐recommended blood glucose or blood pressure targets. However, the management of other cardiometabolic risk factors, including serum lipids and urinary protein (target attainment by fewer than 50% of patients), could be improved.
The implications: Our findings could inform initiatives for improving the secondary prevention of stroke in general practice.
Stroke is a leading cause of death and disability in Australia1 and around the world.2,3 Many people who survive stroke or transient ischaemic attacks (TIAs) have several other medical conditions (eg, diabetes) or risk factors (eg, hypertension)4 that increase the risk of further vascular events if not well managed.5 Continuity of stroke care for people in the community is important. For example, seeing a general practitioner or neurologist shortly after hospitalisation with stroke is associated with increased use of secondary prevention medications.6 Moreover, it is estimated that increasing the number of encounters with general practitioners by 20%, together with more intensive management, could increase the proportion of patients with good blood pressure control from 45% to 66%.7
For secondary prevention of stroke, clinical guidelines emphasise controlling risk factors with routine comprehensive risk factor assessments and appropriate prescribing;8,9,10 more than 80% of strokes could be averted by effective management of cardiometabolic risk factors.11 Despite the increase in the uptake of therapies for secondary prevention of stroke in Australia, long term post‐stroke treatment goals are not being attained.4,12 Moreover, people who survive stroke report unmet long term needs (two or more years after stroke) related to post‐acute care and secondary prevention.13 We recently reported that survival after stroke or TIA in Australia improved after the introduction of financial incentives for general practitioners to develop chronic disease management plans, which often include risk factor assessment and management.14 However, information about the components of general practitioner risk factor management care, and about whether this care is optimal for the secondary prevention of stroke or TIA, is limited.
Real world general practice data can provide insights into the delivery of long term care in the community for people after stroke. Unlike government administrative databases (ie, pharmaceutical, Medicare, hospitals data), primary care data can provide detailed information on post‐acute care, including the management of risk factors and other medical conditions.15 We evaluated the management of people after stroke or TIA in general practices in three Victorian primary health networks, with the aim of identifying factors associated with the quality of risk factor management.
Methods
We undertook a retrospective observational study of people admitted to hospital in Victoria during 2014–2018 with stroke or TIA. We analysed linked person‐level Australian Stroke Clinical Registry (AuSCR)16 data and routinely extracted general practice data for three primary health networks in Victoria (Eastern Melbourne, South Eastern Melbourne, Gippsland), which together serve about 3.3 million people.17 People with clinical diagnoses of stroke or TIA were identified in the AuSCR, a national registry for monitoring care and patient outcomes after acute stroke or TIA.16 General practice encounters after hospital discharge were identified in general practice data collected by Outcome Health (https://www.outcomehealth.org.au) using the POLAR platform15 with the permission of the primary health networks as data custodians.
We included data for adults (18 years or older) admitted to hospital with stroke or TIA and registered in AuSCR during 1 January 2014 – 31 December 2018, and who were discharged home or to rehabilitation after the acute admission. Our observation period was the first year of the chronic phase of stroke (seven to eighteen months after the acute event), as all care is usually managed by general practitioners during this period. People who died before the observation period were not included. We also excluded people who died during the observation period, as they may not have been reviewed for risk factors within the one‐year cycle usually recommended by guidelines.8,9,10 To optimise identification of regular general practitioners, we limited our analysis to people who had visited the same general practice at least twice during the observation period. The number of encounters was defined as the number of days on which a general practitioner service was recorded during the observation period. We excluded encounters with solely administrative purposes (eg, to collect medical certificates), surgical procedures (eg, diagnostic biopsy, abscess incision), or women's health (related to pregnancy or birth control), as these services are unrelated to chronic disease management. To avoid overcounting encounters, multiple general practice encounters within a period of two weeks were treated as single encounters as they were probably related to the same health event. We did not include people discharged to residential aged care because of the complexity of general practice models for aged care facility residents.
Data standardisation
Extracted general practice data were standardised using machine learning models in POLAR; diagnosis fields were mapped to SNOMED CT, Australian extension (https://www.snomed.org), prescription data to the World Health Organization Anatomical Therapeutic Chemical (ATC) classification system,18 and pathology data to a clinical model relevant to general practice (derived by manually classifying the codes from several pathology providers).19
Patient management in general practice
We estimated the proportions of patients:
- for whom assessments of cardiometabolic risk factors were recorded in general practice records, including blood pressure, serum lipid level, glycated haemoglobin (HbA1c) or fasting plasma glucose level, and kidney function (estimated glomerular filtration rate [eGFR] or albumin–creatinine ratio);
- for whom recorded cardiometabolic risk factor values were within target ranges;8,9,10 and
- for whom prescribing of secondary prevention medications (blood pressure‐lowering, lipid‐lowering, antithrombotic, glucose‐lowering agents) was recorded, both overall and for people with conditions for which prescribing would be appropriate.
Blood pressure assessment data were obtained from records of point‐of‐care measurements, and other risk factor assessment data from pathology test results. The prescribing of recommended medication classes was ascertained from medium level ATC codes (for pharmacological or therapeutic subgroup)18 in electronic medical records. For people with recorded risk factor assessments, attainment of metabolic targets was based on the most recent recorded assessment of each risk factor during the observation period. Target values for cardiometabolic risk factors were based on recommendations in clinical guidelines and reference values in pathology guidelines current in Australia during 2014–2018 (Box 1).8,9,10 Measures of kidney function were excluded from analyses of target attainment because the required information on disease progression and patient circumstances was not available. To assess whether care processes and risk factor targets were appropriate for people with specific conditions,8,9,10 we investigated subgroups of patients with clinical indications for risk factor assessment or prescribing, such as records of blood glucose level assessments or the prescribing of glucose‐lowering agents for people with diabetes.
Patient characteristics
Information regarding demographic characteristics (age, sex, residential postcode), stroke type, stroke history, and survival status was obtained from the AuSCR. Postcode‐based residential socio‐economic position was based on the Index of Relative Socioeconomic Advantage and Disadvantage (IRSAD, by quintile).20 Based on residential postcode, the geographic location of registrants was defined as either regional or metropolitan. Information on other medical conditions was based on diagnoses to the end of the observation period, coded using SNOMED CT, Australian extension, and converted to International Classification of Diseases, tenth revision, Australian modification (ICD‐10‐AM) codes with the SnoMAP online tool.21
Statistical analysis
As clinicians are required to document assessments and prescribing during consultations with patients, we assumed that activities not recorded were not undertaken. Our analyses were therefore based on information in general practice records, with the assumption that the absence of a recorded risk factor assessment or treatment indicated “no assessment” for the risk factor or “no prescription” of the medication. This approach is consistent with national audit procedures and medico‐legal reviews.
Patient characteristics are summarised as descriptive statistics, and the statistical significance of differences between those with or without recorded risk factor assessments were assessed in Kruskal–Wallis or χ2 tests. Factors associated with the numbers of risk factors assessed and medication classes prescribed during the observation period were determined in stepwise multivariable Poisson regression models. We report incidence rate ratios (IRRs) with 95% confidence intervals (CIs); that is, the incidence of one more risk factor being assessed or one more class of medications being prescribed. Factors associated with the attainment of risk factor targets were assessed in stepwise multivariable logistic regression models; we reported odds ratios (ORs) with 95% CIs; that is, the likelihood of attaining target values for a risk factor.
For stepwise regression, initial models included all patient characteristics, the quadratic term for age (to test for a non‐linear association), the number of general practice encounters during the observation period (or the number of times a risk factor was assessed for analyses of target attainment), and a cluster sandwich estimator (to account for correlation within practices). Manual bi‐directional, iterative removal and restoration of variables was used to achieve parsimonious models. Age, sex, and number of encounters (fixed in all models) and variables for which P < 0.10 were retained in final models.
In sensitivity analyses, attainment of risk factor targets was based on randomly selected assessments of the risk factor, rather than the final recorded assessment. All analyses were undertaken in Stata/SE 16. P < 0.05 (two‐sided) was deemed statistically significant.
Ethics approval
The human research ethics committee of Monash University approved the study (CF13/1303–2013000641).
Results
During the observation period, 4476 AuSCR registrants had at least one encounter with one of the 383 general practices in the three primary health networks covered by POLAR; 3376 eligible registrants (75.4%) were included in our analysis (Supporting Information, figure 1). The median number of encounters during the observation period was six (interquartile range [IQR], 4–8; maximum number, 15). The median age at stroke onset was lower for registrants included in our analysis (73.9 [IQR, 64.4–81.9] years) than for those who were not (76.5 [IQR, 65.1–84.8] years), and the proportion of women was smaller (1465, 43.4% v 553, 50.3%); the proportion of included patients who had been hospitalised with TIAs was larger (737, 21.8% v 175, 15.9%), as was the proportion living in areas in the two least disadvantaged IRSAD quintiles (2143, 63.2% v 626, 56.9%) (Supporting Information, table 1).
Assessment of risk factors
During the observation period, no cardiometabolic risk factors had been assessed for 303 patients (9.0%), one risk factor for 789 (23.7%), two risk factors for 695 (20.6%), three risk factors for 597 (17.7%), and all four risk factors for 992 patients (29.4%). The median number of risk factors assessed was two per patient (IQR, 1–3). Blood pressure was assessed in 2718 patients (80.5%), serum lipids in 1830 (54.2%), blood glucose in 1708 (50.6%), and urinary protein in 1685 patients (49.9%) (Box 2).
The proportion of women was smaller for patients assessed for at least one risk factor than for those not assessed for risk factors (1312 of 3073, 42.7% v 153 of 303, 50.5%). The proportions living in regional locations (377, 12.4% v 22, 7.5%) or who had hypertension (60.1% v 38.3%), diabetes (30.0% v 14.9%), dyslipidaemia (40.0% v 20.1%), or cardiovascular disease (21.0% v 12.5%) were larger for people assessed for risk factors than for those who were not assessed (Box 3).
The incidence of risk factors being assessed was lower for patients aged 60 years or younger (IRR, 0.96, 95% CI 0.90–1.01; not statistically significant) and those older than 80 years of age (IRR, 0.91; 95% CI, 0.87–0.95) than for those aged 61–80 years (quadratic term: P < 0.001). The incidence of risk factors being assessed was lower for women (IRR, 0.91; 95% CI, 0.87–0.95) and for people with a history of stroke (IRR, 0.92; 95% CI, 0.87–0.96), who were discharged to rehabilitation (IRR, 0.92; 95% CI, 0.88–0.95), or who had dementia (IRR, 0.89; 95% CI, 0.81–0.98); the incidence was higher for people with hypertension (IRR, 1.07; 95% CI, 1.02–1.12), diabetes (IRR, 1.30; 95% CI, 1.25–1.35), or dyslipidaemia (IRR, 1.08; 95% CI, 1.04–1.12) (Box 4).
Prescribing of medications
A total of 2949 patients (87.4%) were prescribed recommended medications; 341 (10.1%) were prescribed all four classes of prevention medications. The most prescribed medications were lipid‐lowering (2427 patients, 71.9%) and blood pressure‐lowering agents (2363, 70.0%); 548 of 653 people who had been hospitalised with ischaemic stroke or TIA and atrial fibrillation (83.9%) had been prescribed anticoagulant agents (Box 5). The incidence of prescribing of more classes of prevention medications was lower for patients aged 60 years or younger (IRR, 0.92; 95% CI, 0.88–0.97) and those older than 80 years (IRR, 0.96; 95% CI, 0.92–0.997) than for patients aged 61–80 years (quadratic term: P < 0.001). The incidence was also lower for women (IRR, 0.95; 95% CI, 0.91–0.98) and people with dementia (IRR, 0.88; 95% CI, 0.78–0.98) or a history of anxiety or depression (IRR, 0.95; 95% CI, 0.92–0.99). People who had been hospitalised with ischaemic stroke or TIA, or who had diabetes, hypertension, dyslipidaemia, atrial fibrillation, cardiovascular disease, or liver disease were more likely to be prescribed more classes of prevention medications than people without these conditions (Box 4).
Attainment of risk factor targets
Blood glucose targets were achieved by 1346 of 1708 patients assessed for this risk factor (78.8%), blood pressure targets by 1935 of 2717 (71.2%); and serum lipid targets by 765 of 1830 (41.8%) (Box 6). Sensitivity analyses based on randomly selected risk factor assessments rather than the final assessment yielded similar results (data not shown).
The likelihood of attaining targets increased with age for serum lipid (per five years: OR, 1.17; 95% CI, 1.07–1.28) and urinary protein assessments (OR, 1.31; 95% CI, 1.17–1.48). Attainment of blood pressure (OR, 0.59; 95% CI, 0.49–0.71) and blood glucose targets (OR, 0.75; 95% CI, 0.58–0.98) was less likely for people with a history of hypertension. People with diabetes were more likely to attain urinary protein targets (OR, 3.16; 95% CI, 2.46–4.04). People with a history of atrial fibrillation were more likely to achieve blood pressure and lipid targets, people with dyslipidaemia lipid targets, and people with kidney disease urinary protein targets; patients with a history of cardiovascular disease or dementia were less likely to achieve blood glucose targets (Supporting Information, table 5).
Discussion
In our analysis of the provision of recommended risk factor management care processes in Victorian general practices for people after stroke or TIA, we found that the assessment and management of blood pressure and blood glucose is satisfactory. However, some aspects of cardiometabolic risk factor management for the secondary prevention of stroke could be improved. A considerable proportion of patients with clinical indications for assessing serum lipid levels (those who had experienced an ischaemic stroke or TIA) or urinary protein (people with diabetes) had not been assessed for these risk factors. This finding may explain why considerable proportions of patients had not been prescribed medications for managing these factors. Although the proportions of people who attained risk factor‐related targets were generally larger than in an earlier study of general practice care for people after stroke in Australia,12 our findings reflect the challenges of managing risk factors, particularly in people who survive stroke who have other related medical conditions.
Data on the quality of the long term management in general practice of people who have had strokes are sparse, both in Australia and overseas. Our findings regarding the prescribing of preventive medications complement those of a study of the management of people aged 30 years or older with coronary heart disease in 438 Australian general practices over two years; 72% of patients were prescribed lipid‐lowering medications and 50% antiplatelet agents.22 However, the proportions of patients whose blood pressure (95%) or serum lipid levels (75%) were assessed were larger than in our study, possibly because of the longer follow‐up period. Similarly, our estimates differed considerably from those of an audit of records of risk factor assessment in people at risk of cardiovascular disease (based on absolute global risk) who attended 268 general practices across Europe over a 15‐month period: blood pressure was assessed in 93% of patients, serum lipid levels in 84%, and blood glucose in 76%, each proportion larger than in our analysis.23
The non‐linear relationship between age and the likelihood of prescribing of prevention medications we report is consistent with the findings of an Australian audit in 2000 of blood glucose‐ lowering and lipid‐lowering medication prescribing for primary stroke prevention by 321 general practitioners.24 Findings of a non‐linear association of age with the likelihood of risk factor assessment contrast with the positive linear associations found by an earlier study of secondary prevention care for people with coronary heart disease.22 However, our findings that risk factor assessment was less likely for women and more likely for people with certain medical conditions are consistent with those of the earlier study.
Limitations
Our analysis included data for a large sample of people attending a large number of Australian general practices. Further, linkage with the national stroke registry ensured reliable identification of people with clinical diagnoses of stroke or TIA in general practice data. We provide the first example of the feasibility and value of linking national stroke registry data with general practice data. The merged records provide considerably more information than routine claims data for assessing the long term management in the community of people who have had strokes.
People may have received care during the observation period in general practices other than those covered by POLAR, or elsewhere. However, all included patients fulfilled the standard definition of “active patients”; that is, three or more encounters within a two‐year window,25 as confirmed by a post hoc analysis of the number of encounters during the first two years after discharge from the index stroke or TIA hospitalisation (data not shown). Our findings may not be generalisable to all Australian practices, as we analysed data from only three urban Victorian primary health networks. However, the age and sex distributions of the included patients were similar to those of a nationally representative cohort.16 As general practitioners may not record all risk factor assessments or medications prescribed, our assumption that “no record” meant “not undertaken” may have influenced our findings. Moreover, attainment of risk factor targets was based on single (final) assessments, which may not reflect long term control; however, our findings were similar when we instead used random assessments for this analysis.
Using risk factor target values to evaluate management is not ideal, as targets should be individualised for each patient. For example, HbA1c target levels often depend on the person's age and the level of progression and complications of diabetes. We used a target of no more than 53 mmol/mol HbA1c for practical reasons, as this level is generally used to define good blood glucose control.10 Our findings may also be limited by the quality of data captured by and retrievable from general practice records (diagnoses, point‐of‐care assessments) and the completeness of health information transferred to practices (pathology test results). We did not have data on lifestyle risk factors for stroke, such as current smoking status and diet.
Conclusion
Information about the ongoing management of risk factors in general practice are crucial for informing initiatives for the secondary prevention of stroke and TIA. The implications of suboptimal management of cardiometabolic risk factors for people after stroke are considerable, including increased risks of emergency department presentations and unplanned hospitalisations because of secondary adverse events,5 and death.14 We identified areas that require further attention from general practitioners with the aim of improving the management of people after stroke or TIA. Effective monitoring of cardiometabolic risk factors will enable general practitioners to optimise care for people who need careful attention to avert adverse secondary events.
Box 1 – Target values for risk factor management, based on Australian clinical guidelines8,9,10
Risk factor |
Target values |
||||||||||||||
|
|||||||||||||||
Blood pressure |
Systolic blood pressure ≤ 140 mmHg and diastolic blood pressure ≤ 90 mmHg |
||||||||||||||
Serum lipids |
HDL‐cholesterol > 1.0 mmol/L, LDL‐cholesterol < 2.0 mmol/L, total cholesterol < 4.0 mmol/L; or |
||||||||||||||
Blood glucose |
HbA1c ≤ 53 mmol/mol; or |
||||||||||||||
|
|||||||||||||||
HbA1c = glycated haemoglobin; HDL = high‐density lipoprotein; LDL = low‐density lipoprotein. |
Box 2 – Proportions of patients for whom assessments in general practice of cardiometabolic risk factors were recorded (7–18 months after acute stroke or transient ischaemic attack)*
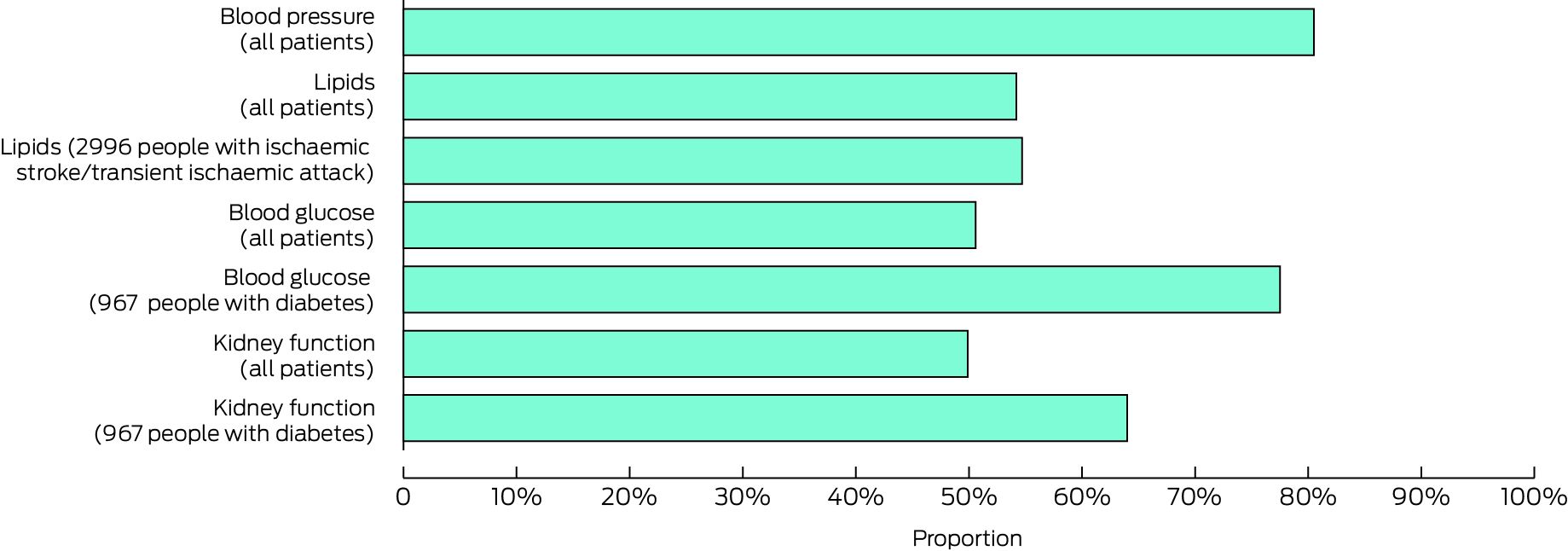
* Data for this graph are included in the Supporting Information, table 2.
Box 3 – Patient characteristics, by assessment of risk factors (none or at least one)
Characteristic |
All patients |
No risk factors assessed |
At least one risk factor assessed |
P* |
|||||||||||
|
|||||||||||||||
All patients |
3376 |
303 [9.0%] |
3073 [91.0%] |
|
|||||||||||
Age at stroke onset (years), median (IQR) |
73.9 (64.4–81.9) |
74.0 (61.6–84.4) |
73.9 (64.7–81.7) |
0.90 |
|||||||||||
Sex (women) |
1465 (43.4%) |
153 (50.5%) |
1312 (42.7%) |
0.009 |
|||||||||||
Socio‐economic advantage (IRSAD) |
|
|
|
0.54 |
|||||||||||
Quintile 1 (least advantaged) |
335 (9.9%) |
36 (11.9%) |
299 (9.7%) |
|
|||||||||||
Quintile 2 |
274 (8.1%) |
19 (6.3%) |
255 (8.3%) |
|
|||||||||||
Quintile 3 |
633 (18.8%) |
57 (18.8%) |
576 (18.7%) |
|
|||||||||||
Quintile 4 |
841 (24.9%) |
71 (23.4%) |
770 (25.1%) |
|
|||||||||||
Quintile 5 (most advantaged) |
1293 (38.3%) |
120 (39.6%) |
1173 (38.2%) |
|
|||||||||||
Geographic location (regional) |
399 (12.0%) |
22 (7.5%) |
377 (12.4%) |
0.012 |
|||||||||||
Stroke type |
|
|
|
0.19 |
|||||||||||
Ischaemic |
2259 (66.9%) |
207 (68.3%) |
2052 (66.8%) |
|
|||||||||||
Intracerebral haemorrhage |
290 (8.6%) |
31 (10.2%) |
259 (8.4%) |
|
|||||||||||
Transient ischaemic attack |
737 (21.8%) |
54 (17.8%) |
683 (22.3%) |
|
|||||||||||
Undetermined |
90 (2.7%) |
11 (3.6%) |
79 (2.6%) |
|
|||||||||||
Prior stroke |
661 (20.1%) |
67 (22.3%) |
594 (19.9%) |
0.31 |
|||||||||||
Discharged to rehabilitation |
1153 (34.2%) |
134 (44.2%) |
1019 (33.2%) |
< 0.001 |
|||||||||||
Other medical conditions |
|
|
|
|
|||||||||||
Hypertension |
1962 (58.1%) |
116 (38.3%) |
1846 (60.1%) |
< 0.001 |
|||||||||||
Diabetes |
967 (28.6%) |
45 (14.9%) |
922 (30.0%) |
< 0.001 |
|||||||||||
Atrial fibrillation |
710 (21.0%) |
47 (15.5%) |
663 (21.6%) |
0.013 |
|||||||||||
Dyslipidaemia |
1291 (38.2%) |
61 (20.1%) |
1230 (40.0%) |
< 0.001 |
|||||||||||
Cardiovascular disease† |
682 (20.2%) |
38 (12.5%) |
644 (21.0%) |
< 0.001 |
|||||||||||
Obesity |
61 (1.8%) |
5 (1.7%) |
56 (1.8%) |
0.83 |
|||||||||||
Kidney disease |
286 (8.5%) |
20 (6.6%) |
266 (8.7%) |
0.22 |
|||||||||||
Liver disease |
167 (5.0%) |
9 (3.0%) |
158 (5.1%) |
0.10 |
|||||||||||
Anxiety or depression |
867 (25.7%) |
68 (22.4%) |
799 (26.0%) |
0.18 |
|||||||||||
Dementia |
125 (3.7%) |
12 (4.0%) |
113 (3.7%) |
0.80 |
|||||||||||
History of smoking |
84 (2.5%) |
7 (2.3%) |
77 (2.5%) |
0.85 |
|||||||||||
|
|||||||||||||||
IQR = interquartile range; IRSAD = Index of Relative Socioeconomic Advantage and Disadvantage.20 * No risk factors assessed v at least one risk factor assessed. † Other than stroke. |
Box 4 – Factors associated with being assessed for risk factors or prescribed prevention medications in general practice (7–18 months after acute stroke or transient ischaemic attack; 3376 people): multivariable Poisson regression analyses*
|
Incidence rate ratio (95% CI) |
||||||||||||||
Characteristic |
Number of risk factors assessed† |
Number of medications classes prescribed‡ |
|||||||||||||
|
|||||||||||||||
Age group at stroke onset (years) |
|
|
|||||||||||||
60 or younger |
0.96 (0.90–1.01) |
0.92 (0.88–0.97) |
|||||||||||||
61–80 |
1 |
1 |
|||||||||||||
Older than 80 |
0.91 (0.87–0.95) |
0.96 (0.92–0.997) |
|||||||||||||
Sex (women) |
0.91 (0.87–0.95) |
0.95 (0.91–0.98) |
|||||||||||||
Stroke type: ischaemic stroke/transient ischaemic attack |
— |
1.41 (1.31–1.51) |
|||||||||||||
Prior stroke |
0.92 (0.87–0.96) |
— |
|||||||||||||
Discharge to rehabilitation |
0.92 (0.88–0.95) |
— |
|||||||||||||
Other medical conditions |
|
|
|||||||||||||
Hypertension |
1.07 (1.02–1.12) |
1.29 (1.23–1.34) |
|||||||||||||
Diabetes |
1.30 (1.25–1.35) |
1.33 (1.29–1.38) |
|||||||||||||
Atrial fibrillation |
— |
1.14 (1.10–1.18) |
|||||||||||||
Dyslipidaemia |
1.08 (1.04–1.12) |
1.09 (1.05–1.13) |
|||||||||||||
Cardiovascular disease |
— |
1.11 (1.07–1.15) |
|||||||||||||
Liver disease |
— |
1.07 (1.01–1.14) |
|||||||||||||
Dementia |
0.89 (0.81–0.98) |
0.88 (0.78–0.98) |
|||||||||||||
Anxiety or depression |
— |
0.95 (0.92–0.99) |
|||||||||||||
|
|||||||||||||||
CI = confidence interval. * Manual backward stepwise elimination; the initial model included all variables in Box 3, the quadratic term for age, and clustering effect within 383 general practices. † Blood pressure, serum lipids, blood glucose, and urinary protein. ‡ Blood pressure‐lowering, lipid‐lowering, antithrombotic, and glucose‐lowering agents. |
Box 5 – Proportions of patients for whom protective medications were prescribed in general practice (7–18 months after acute stroke or transient ischaemic attack)*
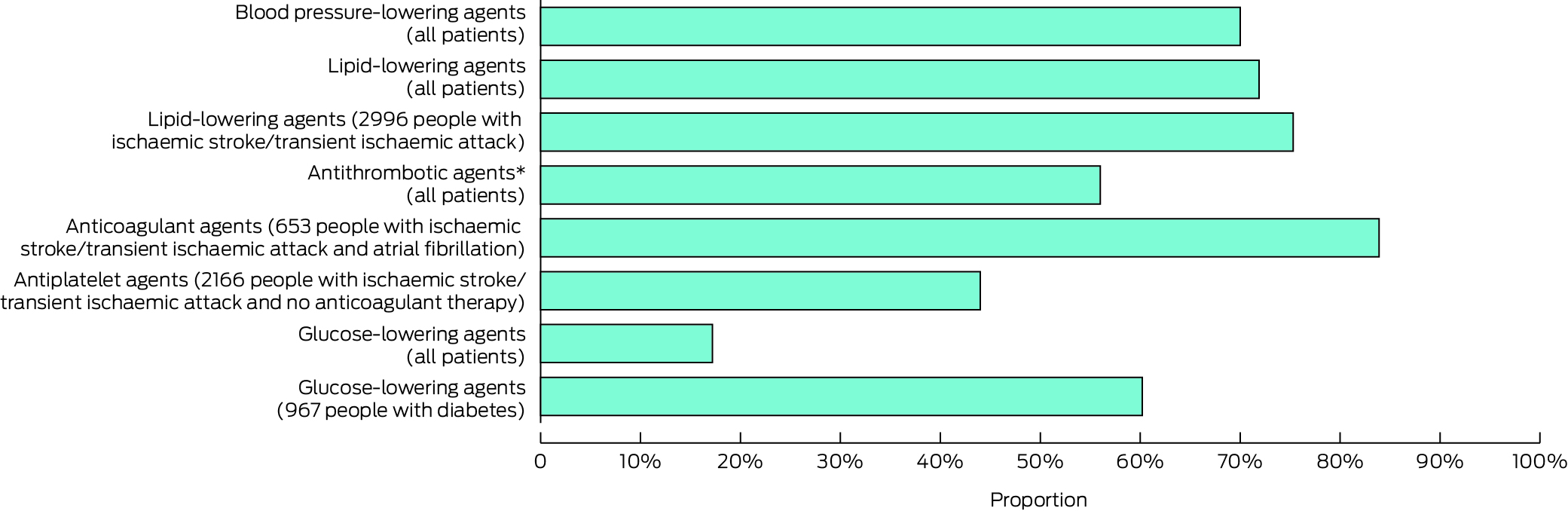
* Data for this graph are included in the Supporting Information, table 3.† Anticoagulant or antiplatelet agents.
Box 6 – Proportion of patients who attained risk factor targets 7–18 months after acute stroke or transient ischaemic attack, based on final recorded assessments of risk factor*
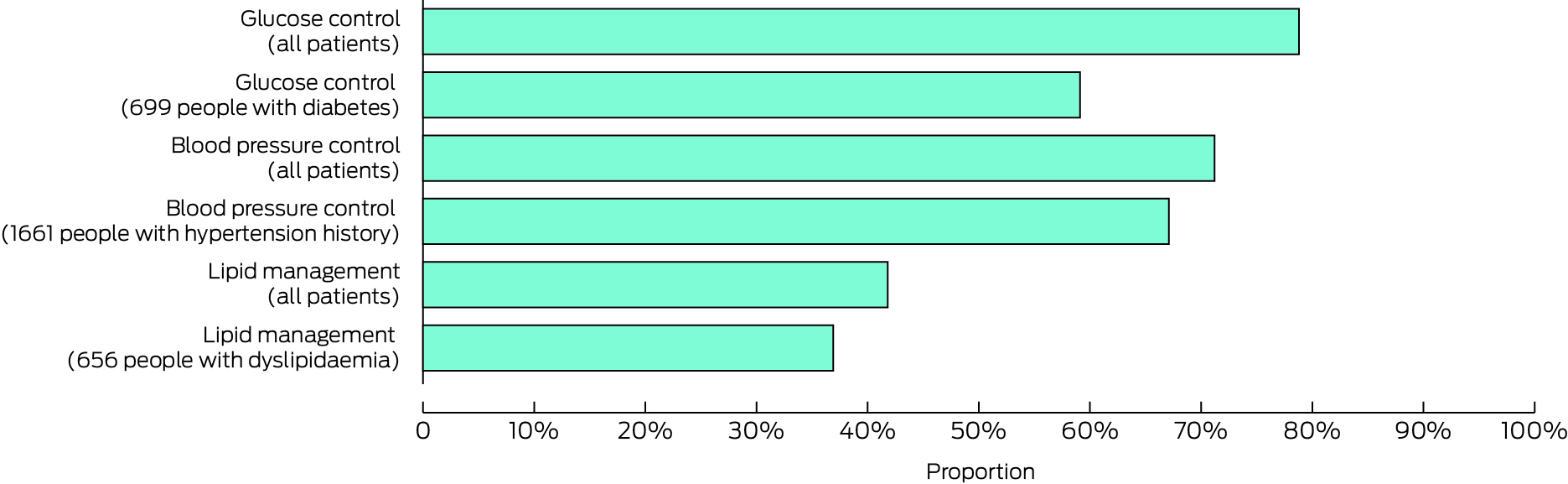
* Data for this graph are included in the Supporting Information, table 4.
Received 7 August 2023, accepted 26 March 2024
Abstract
Objective: To evaluate the management in Victorian general practice of people who have been hospitalised with stroke or transient ischaemic attacks (TIA).
Study design: Retrospective observational study; analysis of linked Australian Stroke Clinical Registry (AuSCR) and general practice data.
Setting: 383 general practices in the Eastern Melbourne, South Eastern Melbourne, and Gippsland primary health networks (Victoria), 1 January 2014 – 31 December 2018.
Participants: Adults who had been hospitalised with acute stroke or TIA and had at least two encounters with the same general practice during the observation period (7–18 months after the acute event).
Main outcome measures: Assessment of cardiometabolic risk factors (blood pressure, serum lipids, blood glucose, urinary protein); prescribing of guideline‐recommended prevention medications (blood pressure‐, lipid‐, or glucose‐lowering, antithrombotic agents); attainment of guideline targets for cardiometabolic risk factors at final assessment during observation period.
Results: During 2014–2018, 3376 eligible AuSCR registrants (1465 women, 43.4%) had at least two encounters with one of the 383 general practices during the observation period; median age at stroke onset was 73.9 (interquartile range, 64.4–81.9) years, 737 events were TIAs (21.8%). Blood pressure was assessed in 2718 patients (80.5%), serum lipids in 1830 (54.2%), blood glucose in 1708 (50.6%). Prevention medications were prescribed for 2949 patients (87.4%), including lipid‐lowering (2427, 71.9%) and blood pressure‐lowering agents (2363, 70.0%). Blood glucose targets had been achieved by 1346 of 1708 patients assessed for this risk factor (78.8%), blood pressure targets by 1935 of 2717 (71.2%), and serum lipid targets by 765 of 1830 (41.8%). The incidence of having risk factors assessed was lower among patients aged 60 years or younger (incidence rate ratio [IRR], 0.97; 95% confidence interval [CI], 0.92–1.03) and those over 80 years of age (IRR, 0.92; 95% CI 0.88–0.97) than for those aged 61–80 years, and for women (IRR, 0.91; 95% CI, 0.87–0.95) and people with dementia (IRR, 0.89; 95% CI, 0.81–0.98). The likelihood of having classes of prevention medication prescribed was lower for patients aged 60 years or younger (IRR, 0.92; 95% CI, 0.88–0.97) and those over 80 years of age (IRR, 0.96; 95% CI, 0.92–0.997) than for patients aged 61–80 years, and for women (IRR, 0.95; 95% CI, 0.91–0.98) and people with dementia (IRR, 0.88; 95% CI, 0.78–0.98).
Conclusions: The general practice management of people who have been hospitalised with stroke or TIA could be improved. Effective monitoring of cardiometabolic risk factors will enable general practitioners to optimise care for people who need careful attention to prevent adverse secondary events.